class-incremental incremental distiling learning
Contrastive Learning for Representation Degeneration Problem in Sequential Recommendation
[TOC] > [Qiu R., Huang Z., Ying H. and Wang Z. Contrastive learning for representation degeneration problem in sequential recommendation. WSDM, 2022.] ......
Achieving a Better Stability-Plasticity Trade-off via Auxiliary Networks in Continual Learning论文阅读笔记
## 摘要 连续学习过程中的稳定性-可塑性权衡是一个重要的问题。作者提出了Auxiliary Network Continual Learning (ANCL),通过auxiliary network提高了模型的可塑性。 ## 方法 ### The Formulation of Auxiliary ......
火山引擎DataLeap的Catalog系统搜索实践(三):Learning to rank与后续工作
Learning to rank Learning to rank主要分为数据收集,离线训练和在线预测三个部分。搜索系统是一个Data-driven system,因此火山引擎DataLeap的Catalog系统设计之初就需要考虑数据收集。收集的数据可以用来评估和提升搜索的效果。数据收集和在线预测前 ......
Reinforcement Learning之Q-Learning - Python实现
- **算法特征** ①. 以真实reward训练Q-function; ②. 从最大Q方向更新policy $\pi$ - **算法推导** **Part Ⅰ: RL之原理** 整体交互流程如下, 定义策略函数(policy)$\pi$, 输入为状态(state)$s$, 输出为动作(action ......
Incrementer:Transformer for Class-Incremental Semantic Segmentation with Knowledge Distillation Focusing on Old Class论文阅读笔记
## 摘要 目前已有的连续语义分割方法通常基于卷积神经网络,需要添加额外的卷积层来分辨新类别,且在蒸馏特征时没有对属于旧类别/新类别的区域加以区分。为此,作者提出了基于Transformer的网络incrementer,在学习新类别时只需要往decoder中加入对应的token。同时,作者还提出了对 ......
WebSocket-Learning-1
#### WebSocket ##### 1.什么是WebSocket >> WebSocket 是一种通讯协议,目标就是替代XmlHttpRequest 和长期轮询的解决方案。应用在实时弹幕、消息推送、棋牌游戏、聊天等需要及时通讯的场景。 ##### 2.WebSocket 连接有两个阶段 >>握 ......
强化学习基础篇[2]:SARSA、Q-learning算法简介、应用举例、优缺点分析
# 强化学习基础篇[2]:SARSA、Q-learning算法简介、应用举例、优缺点分析 # 1.SARSA SARSA(State-Action-Reward-State-Action)是一个学习马尔可夫决策过程策略的算法,通常应用于机器学习和强化学习学习领域中。它由Rummery 和 Niran ......
Machine Learning 【note_02】
# note_02 Keywords: Classification, Logistic Regression, Overfitting, Regularization ## 1 Motivation Classification: - "binary classification": $y$ ca ......
Machine Learning 【note_01】
Declaration (2023/06/02): This note is the first note of a series of machine learning notes. At present, the main learning resource is *the 2022 Andre ......
论文阅读 | Learn from Others and Be Yourself in Heterogeneous Federated Learning
**在异构联邦学习中博采众长做自己** 代码:https://paperswithcode.com/paper/learn-from-others-and-be-yourself-in **摘要** 联邦学习中有异质性问题和灾难性遗忘。首先,由于非I.I.D(相同独立分布)数据和异构体系结构,模型在 ......
April 2023-Memory-efficient Reinforcement Learning with Value-based Knowledge Consolidation
本文基于深度q网络算法提出了记忆高效的强化学习算法来缓解这一问题。通过将目标q网络中的知识整合Knowledge Consolidation到当前q网络中,所提算法减少了遗忘并保持了较高的样本效率。 ......
零样本学习(Zero-shot Learning)
零样本学习是一种机器学习的问题设置,其中模型可以对从未在训练过程中见过的类别的样本进行分类,使用一些形式的辅助信息来关联已见和未见的类别。例如,一个模型可以根据动物的文本描述来识别动物,即使它从未见过那些动物的图像。 实现零样本学习有不同的方法,取决于辅助信息的类型和学习方法。以下是一些例子: 一种 ......
事件抽取论文综述-A Survey on Deep Learning Event Extraction: Approaches and Applications
A Survey on Deep Learning Event Extraction: Approaches and Applications 1)发表信息: https://arxiv.org/abs/2107.02126 Qian Li, Jianxin Li, Member, IEEE, Ji ......
[论文速览] MAGE@MAsked Generative Encoder to Unify Representation Learning and Image Synthesis
## Pre title: MAGE: MAsked Generative Encoder to Unify Representation Learning and Image Synthesis accepted: CVPR2023 paper: https://arxiv.org/abs/221 ......
[论文阅读] Few-shot Font Generation by Learning Style Difference and Similarity
## Pre title: Few-shot Font Generation by Learning Style Difference and Similarity accepted: Arxiv 2023 paper: https://arxiv.org/abs/2301.10008 code: ......
[论文速览] RectifiedFlow@Flow Straight and Fast{colon}Learning to Generate and Transfer Data with Rectified Flow
## Pre title: Flow Straight and Fast: Learning to Generate and Transfer Data with Rectified Flow accepted: ICLR 2023 paper: https://arxiv.org/abs/2209 ......
2023CVPR_Learning a Simple Low-light Image Enhancer from Paired Low-light Instances(PairLLE)无监督
一. motivation 以前的大多数LIE算法使用单个输入图像和几个手工制作的先验来调整照明。然而,由于单幅图像信息有限,手工先验的适应性较差,这些解决方案往往无法揭示图像细节。 二. contribution 1. 提出一个成对低光图像输入(相同内容,不同的曝光度) 2. 在输入之前进行了一个 ......
The Difficulty of Passive Learning in Deep Reinforcement Learning
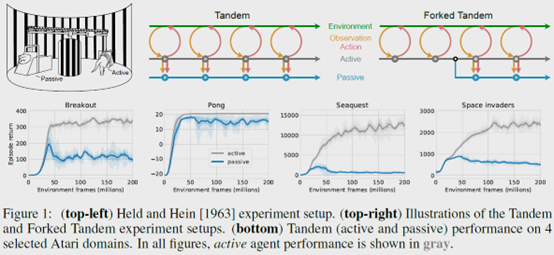 **发表时间:**2021(NeurIPS 2021) **文章要点:**这篇文章提出一个tandem learni ......
learn c++ 参数引用
#include <iostream> struct Role { int hp; int mp; int damage; }; bool Act(Role& Acter,Role& beAct) { beAct.hp -= Acter.damage; return beAct.hp < 0; } ......
Learning with Local and Global Consistency
[TOC] > [Zhou D., Bousquet O., Lal T. N., Weston J. and Scholk\ddot{o}pf B. Learning with local and global consistency. NIPS, 2003.](https://proceedin ......
2023CVPR_Learning a Simple Low-light Image Enhancer from Paired Low-light Instances(PairLIE)
1、nn.ReflectionPad2d 对输入图像以最外围像素为对称轴,做四周的轴对称镜像填充。 大佬链接:(14条消息) torch.nn.ReflectionPad2d()的用法简介_nn.reflectionpad2d(1)_啊菜来了的博客-CSDN博客 # 对四周都填充3行 nn.Refl ......
Learning Affinity from Attention: End-to-End Weakly-Supervised Semantic Segmentation with Transformers概述
0.前言 相关资料: arxiv github 论文解读 论文基本信息: 领域:弱监督语义分割 发表时间: CVPR 2022(2022.3.5) 1.针对的问题 目前主流的弱监督语义分割方法通常首先训练分类模型,基于类别激活图(CAM)或其变种生成初始伪标签;然后对伪标签进行细化作为监督信息训练一 ......
learn c++ 智能指针
#include <iostream> int main() { int* p; { std::unique_ptr<int[]> a{std::make_unique<int[]>(50)}; a[2] = 240; p = a.get(); std::cout << p[2]; } std::c ......
Sep 2022-Prioritized Training on Points that are Learnable, Worth Learning, and Not Yet Learnt
提出了Reducible Holdout Loss Selection (RHOLOSS),一种简单但有原则的技术,近似地选择那些最能减少模型泛化损失的点进行训练 ......
C-pointer Learning
# 基础 ## 指针类型 ### 静态/全局内存 指在内存空间中的全局/静态数据区的指针变量 ### 自动内存 即局部作用域的指针,只有在函数被调用时才创建。 ### 动态内存 在堆区动态创建的指针变量,在不使用时需要即是释放该部分内存空间。 ## 特殊指针 ### NULL指针 在指针变量中,初始 ......
learn c++ 变量作用域
#include <iostream> int a{ 100 }; int main() { int a{160}; { std::cout << a << std::endl; char a = 'a'; std::cout << a << std::endl; std::cout << ::a ......
Off-Policy Deep Reinforcement Learning without Exploration
**发表时间:**2019(ICML 2019) **文章要点:**这篇文章想说在offline RL的setting下,由于外推误差(extrapolation errors)的原因,标准的off-policy算法比如DQN,DDPG之类的,如果数据的分布和当前policy的分布差距很大的话,那就 ......
《AutoInt: Automatic Feature Interaction Learning via Self-Attentive Neural Networks》特征交叉论文阅读
背景 这是一篇利用多头attention机制来做特征交叉的论文 模型结构 AutoInt的模型结构如上图所示,搞模型包含 Embedding Layer、Interacting Layer、Output Layer三个部分,其中Embedding Layer和Output Layer和普通模型没什么 ......
【图像数据增强】Image Data Augmentation for Deep Learning: A Survey
| 原始题目 | Image Data Augmentation for Deep Learning: A Survey | | | | | 中文名称 | 深度学习的图像数据增强:综述 | | 发表时间 | 2022年4月19日 | | 平台 | arXiv | | 来源 | 南京大学 | | 文章 ......